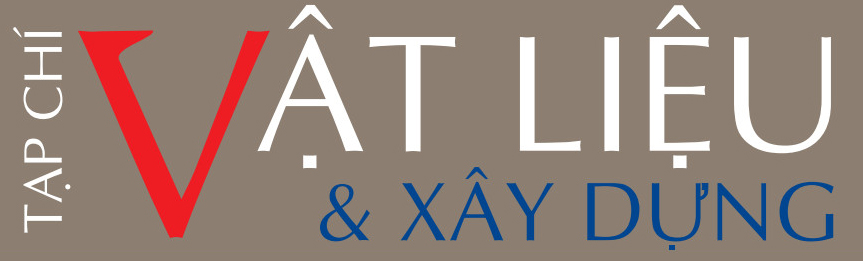
ISSN:
Website: www.jomc.vn
Ứng dụng mô hình học sâu YOLOv8 để kiểm soát hành vi sử dụng phương tiện bảo vệ cá nhân trên công trường xây dựng
Tóm tắt
Trong quá trình thi công tại các công trường xây dựng, việc giám sát các hành vi sử dụng phương tiện bảo vệ cá nhân của công nhân luôn cần sự quan tâm kỹ càng, nhằm tránh xảy ra tai nạn đáng tiếc. Cùng với sự phát triển của khoa học kỹ thuật, công nghệ hình ảnh và các thuật toán trí tuệ nhân tạo liên tục được nghiên cứu và áp dụng vào các công tác ngoài hiện trường. Nghiên cứu này đề xuất một phương pháp ứng dụng mô hình học sâu YOLOv8 – một trong những mô hình tiên tiến nhất – để tự động xác định hành vi sử dụng phương tiện bảo vệ cá nhân của công nhân trên công trường, bao gồm các hành vi sử dụng và không sử dụng phương tiện bảo vệ cá nhân. Kết quả của nghiên cứu cho thấy các phát hiện được cải thiện đáng kể so với những mô hình trước đó, cụ thể độ chính xác với phát hiện mũ bảo hộ, găng tay và khẩu trang lần lượt lên tới 96.6%, 99.6% và 98.2%. Không chỉ vậy, mô hình còn có ý nghĩa rất lớn khi phát hiện được các hành vi không sử dụng phương tiện bảo vệ cá nhân, những trường hợp vốn cần được quan tâm hơn trong công tác giám sát an toàn lao động. Do đó, mô hình học sâu đề xuất có khả năng ứng dụng thực tiễn vào các công trường xây dựng, tăng cường khả năng quản lý, kiểm soát việc sử dụng phương tiện bảo vệ cá nhân, đảm bảo an toàn lao động cho công nhân khi thi công tại các công trường.
Tài liệu tham khảo
- S. Bhole, "Safety problems and injuries on construction site: a review," International Journal of Engineering and Techniques, vol. 2, no. 4, pp. 24-35, 2016.
- S. Ammad et al., "Personal protective equipment in construction, accidents involved in construction infrastructure projects," Solid State Technology, vol. 63, no. 6, pp. 4147-4159, 2020.
- V. S. K. Delhi, R. Sankarlal, and A. Thomas, "Detection of personal protective equipment (PPE) compliance on construction site using computer vision based deep learning techniques," Frontiers in Built Environment, vol. 6, p. 136, 2020.
- N. D. Nath, A. H. Behzadan, and S. G. Paal, "Deep learning for site safety: Real-time detection of personal protective equipment," Automation in Construction, vol. 112, p. 103085, 2020/04/01/ 2020, doi: https://doi.org/10.1016/j.autcon.2020.103085.
- S. Dong, Q. He, H. Li, and Q. Yin, "Automated PPE Misuse Identification and Assessment for Safety Performance Enhancement," in ICCREM 2015, 2015, pp. 204-214.
- H. Zhang, X. Yan, H. Li, R. Jin, and H. Fu, "Real-Time Alarming, Monitoring, and Locating for Non-Hard-Hat Use in Construction," Journal of Construction Engineering and Management, vol. 145, no. 3, p. 04019006, 2019, doi: doi:10.1061/(ASCE)CO.1943-7862.0001629.
- A. Kelm et al., "Mobile passive Radio Frequency Identification (RFID) portal for automated and rapid control of Personal Protective Equipment (PPE) on construction sites," Automation in Construction, vol. 36, pp. 38-52, 2013/12/01/ 2013, doi: https://doi.org/10.1016/j.autcon.2013.08.009.
- J. Seo, S. Han, S. Lee, and H. Kim, "Computer vision techniques for construction safety and health monitoring," Advanced Engineering Informatics, vol. 29, no. 2, pp. 239-251, 2015/04/01/ 2015, doi: https://doi.org/10.1016/j.aei.2015.02.001.
- M.-W. Park, N. Elsafty, and Z. Zhu, "Hardhat-Wearing Detection for Enhancing On-Site Safety of Construction Workers," Journal of Construction Engineering and Management, vol. 141, no. 9, p. 04015024, 2015/09/01 2015, doi: 10.1061/(ASCE)CO.1943-7862.0000974.
- D. Shan, M. Shehata, and W. Badawy, "Hard hat detection in video sequences based on face features, motion and color information," in 2011 3rd International Conference on Computer Research and Development, 11-13 March 2011 2011, vol. 4, pp. 25-29, doi: 10.1109/ICCRD.2011.5763846.
- K. Shrestha, P. P. Shrestha, D. Bajracharya, and E. A. Yfantis, "Hard-Hat Detection for Construction Safety Visualization," Journal of Construction Engineering, vol. 2015, p. 721380, 2015/02/01 2015, doi: 10.1155/2015/721380.
- W. Fang, L. Ding, B. Zhong, P. E. D. Love, and H. Luo, "Automated detection of workers and heavy equipment on construction sites: A convolutional neural network approach," Advanced Engineering Informatics, vol. 37, pp. 139-149, 2018/08/01/ 2018, doi: https://doi.org/10.1016/j.aei.2018.05.003.
- Z. Kolar, H. Chen, and X. Luo, "Transfer learning and deep convolutional neural networks for safety guardrail detection in 2D images," Automation in Construction, vol. 89, pp. 58-70, 2018/05/01/ 2018, doi: https://doi.org/10.1016/j.autcon.2018.01.003.
- L. Ding, W. Fang, H. Luo, P. E. D. Love, B. Zhong, and X. Ouyang, "A deep hybrid learning model to detect unsafe behavior: Integrating convolution neural networks and long short-term memory," Automation in Construction, vol. 86, pp. 118-124, 2018/02/01/ 2018, doi: https://doi.org/10.1016/j.autcon.2017.11.002.
- M. Siddula, F. Dai, Y. Ye, and J. Fan, "Unsupervised Feature Learning for Objects of Interest Detection in Cluttered Construction Roof Site Images," Procedia Engineering, vol. 145, pp. 428-435, 2016/01/01/ 2016, doi: https://doi.org/10.1016/j.proeng.2016.04.010.
- N. D. Nath, T. Chaspari, and A. H. Behzadan, "Single- and multi-label classification of construction objects using deep transfer learning methods," Journal of Information Technology in Construction, vol. 24, no. Special issue Virtual, Augmented and Mixed: New Realities in Construction, pp. 511-526, 2019.
- T. Diwan, G. Anirudh, and J. V. Tembhurne, "Object detection using YOLO: Challenges, architectural successors, datasets and applications," multimedia Tools and Applications, vol. 82, no. 6, pp. 9243-9275, 2023.
- G. Wang, Y. Chen, P. An, H. Hong, J. Hu, and T. Huang, "UAV-YOLOv8: a small-object-detection model based on improved YOLOv8 for UAV aerial photography scenarios," Sensors, vol. 23, no. 16, p. 7190, 2023.
- X. Li et al., "Generalized focal loss: Learning qualified and distributed bounding boxes for dense object detection," Advances in Neural Information Processing Systems, vol. 33, pp. 21002-21012, 2020.
- Z. Zheng, P. Wang, W. Liu, J. Li, R. Ye, and D. Ren, "Distance-IoU loss: Faster and better learning for bounding box regression," in Proceedings of the AAAI conference on artificial intelligence, 2020, vol. 34, no. 07, pp. 12993-13000.